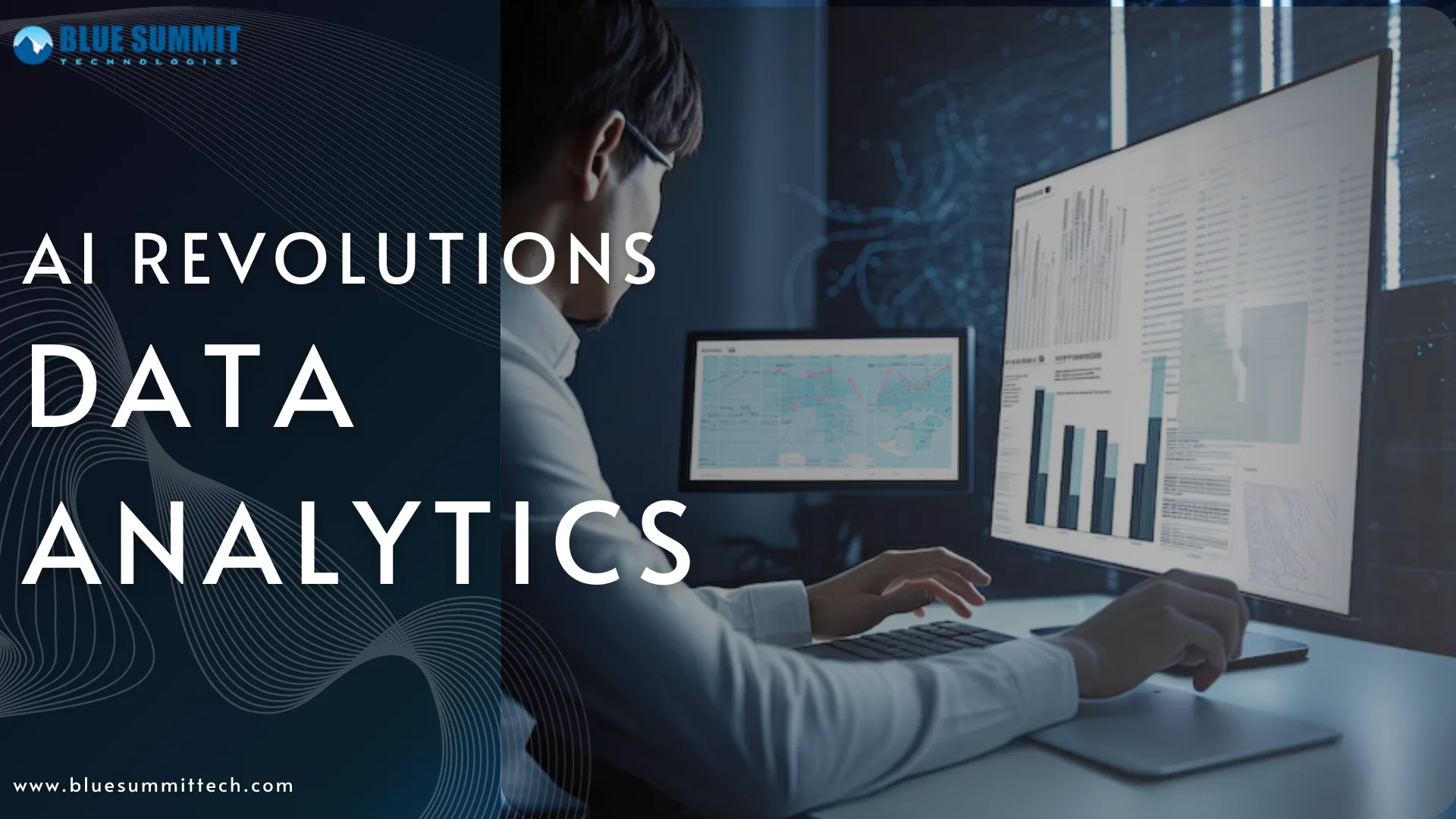
Posted on Friday, May 31st, 2024
Impact of AI on Data Analytics
Artificial Intelligence (AI) is transforming the field of data analytics, offering significant advancements that improve accuracy, efficiency, and predictive capabilities. Businesses are Using AI for data analysis to automate and optimize data processing tasks, allowing for better decision-making and real-time analysis. Artificial intelligence (AI) is a valuable asset across various industries because of its ability to handle large datasets with precision, which reduces human error and speeds up insights. The advantages of AI data analytics are examined in this article, with a focus on how AI can process real-time data, improve data quality, and advance predictive analytics. It also explores the fundamental stages of data preparation, collection, and normalization, emphasizing how crucial sound data management techniques are to maximizing AI's potential in data-driven settings.
Benefits of AI in Data Analytics
Artificial Intelligence (AI) has revolutionized numerous industries, and its impact on data analytics is particularly profound. By leveraging AI, businesses can significantly enhance their data analytics capabilities, leading to improved accuracy and efficiency, real-time data processing and analysis, and enhanced predictive capabilities. These advancements are crucial for making informed decisions, optimizing operations, and maintaining a competitive edge.1. Improved Accuracy and Efficiency
One of the most significant benefits of AI in data analytics is the improvement in accuracy and efficiency. Traditional data analysis methods often rely on manual processes, which are prone to human error and can be time-consuming. AI algorithms, however, can process vast amounts of data with remarkable precision and speed. By automating data cleaning, integration, and analysis tasks, AI ensures higher data quality and reduces the likelihood of errors.For instance, AI-driven tools can identify and correct inconsistencies, duplicates, and anomalies in datasets, leading to more reliable outcomes. This process, known as "AI data quality" enhancement, ensures that the data used for analysis is accurate and up-to-date. Consequently, organizations can make decisions based on solid and reliable data, improving their overall efficiency and effectiveness.
AI can continuously learn and adapt to new data, refining its algorithms and improving performance over time. This iterative learning process allows AI systems to become increasingly accurate in their predictions and analyses, further enhancing their utility in data analytics with AI.
2. Real-Time Data Processing and Analysis
The ability to process and analyze data in real time is another critical advantage of AI in data analytics. In today’s fast-paced business environment, timely insights are crucial for staying competitive. AI data analytics systems can handle streaming data, providing immediate insights and enabling organizations to respond swiftly to changing conditions.Real-time data processing is particularly valuable in industries such as finance, healthcare, and e-commerce, where rapid decision-making is essential. For example, in financial markets, AI algorithms can analyze market trends and execute trades within milliseconds, capitalizing on fleeting opportunities. In healthcare, real-time data analysis can assist in monitoring patient vitals and alerting medical professionals to any critical changes, potentially saving lives. AI’s capacity for real-time data processing also supports dynamic and adaptive decision-making. Businesses can continuously monitor performance metrics, customer behaviors, and market trends, adjusting their strategies and operations as needed. This agility is a significant competitive advantage, allowing organizations to stay ahead of market shifts and customer demands.
3. Enhanced Predictive Capabilities
AI significantly enhances predictive capabilities in data analytics, allowing organizations to anticipate future trends and behaviors with greater accuracy. Predictive analytics involves using historical data to forecast future outcomes, and AI excels in this domain due to its ability to identify complex patterns and relationships within large datasets.a. Machine Learning Models
AI algorithms, such as decision trees, neural networks, and support vector machines, learn from historical data to make accurate predictions. Whether it’s forecasting sales, predicting disease outbreaks, or estimating customer churn, these models provide valuable insights.b. Personalization and Recommendation Systems
E-commerce platforms, streaming services, and social media leverage AI to recommend products, movies, or content tailored to individual preferences. These personalized recommendations enhance user experiences and drive engagement.c. Anomaly Detection
AI algorithms excel at spotting anomalies—unexpected deviations from normal patterns. In fraud detection, for instance, AI can identify unusual credit card transactions or network intrusions.with AI driven data analytics Organizations can unlock unprecedented opportunities. Improved accuracy, real-time insights, and enhanced predictive capabilities empower decision-makers to navigate complex challenges effectively. However, ensuring data quality remains crucial. Organizations must invest in robust data governance practices to maximize the benefits of AI-driven analytics.
Data Collection and Preparation
Data collection and preparation are foundational steps in any data analytics process, and their importance is magnified when integrating AI-driven data analytics. The quality of the data directly impacts the insights and outcomes derived from analytics. Thus, ensuring robust data collection and meticulous preparation is crucial for achieving high AI data quality. This article delves into the various sources of data and the essential processes involved in preparing data for AI-driven analytics.1. Data Sources
AI data analytics thrives on diverse and comprehensive datasets. There are several primary data sources from which data can be collected:i. Databases: Traditional relational databases and modern NoSQL databases are rich sources of structured data. They store vast amounts of information in an organized manner, making it relatively straightforward to query and extract data. Databases are crucial for historical data analysis and ongoing data monitoring.
ii. APIs (Application Programming Interfaces): APIs are increasingly popular for accessing data from various platforms and services. They allow for real-time data collection from external sources such as social media platforms, financial markets, and weather services. APIs provide a standardized way to fetch data, often in real-time, which is essential for dynamic and up-to-date analytics.
iii. Web Scraping: Web scraping involves extracting data from websites. This method is particularly useful for gathering data that is not readily available through APIs or databases. For instance, web scraping can collect data on product prices, customer reviews, and competitive intelligence. However, it’s important to adhere to legal and ethical guidelines when scraping websites.
iv. Internal Systems: Many organizations collect data from their internal systems, such as CRM (Customer Relationship Management) software, ERP (Enterprise Resource Planning) systems, and IoT (Internet of Things) devices. This data is valuable for understanding internal processes, customer interactions, and operational efficiencies.
2. Data Cleaning
Once data is collected, the first step in preparation is data cleaning. This process involves identifying and correcting errors and inconsistencies in the data to improve its quality. AI driven data analytics tools often include features for automated data cleaning, which can significantly enhance AI data quality by reducing the likelihood of errors.i. Error Detection: AI tools can detect common errors such as missing values, duplicates, and outliers. By applying machine learning algorithms, these tools can learn from past data patterns to identify anomalies that may not be immediately apparent.
ii. Correction: After errors are detected, AI algorithms can suggest or automatically apply corrections. For example, missing values can be imputed using statistical methods or machine learning models trained on similar data.
iii. Deduplication: Removing duplicate entries is essential to ensure that analyses are not skewed by redundant data. AI-driven tools can efficiently identify and merge duplicates, preserving data integrity.
3. Data Transformation
Data transformation involves converting data into a suitable format for analysis. This step is crucial for ensuring that data from different sources can be integrated and compared effectively.i. Normalization: Normalizing data means scaling numerical values to a common range, which is particularly important for algorithms that are sensitive to the scale of data. For instance, transforming all numerical values to a range between 0 and 1 can help improve the performance of machine learning models.
ii. Encoding Categorical Data: Many machine learning algorithms require numerical input, so categorical data (e.g., names, types, categories) must be converted into numerical formats. Techniques such as one-hot encoding and label encoding are commonly used for this purpose.
iii. Feature Engineering: This process involves creating new features from existing data that can provide additional insights. For example, combining date and time fields into a single timestamp or extracting the day of the week from a date field can provide a more granular analysis.
4. Data Normalization
Normalization is a specific type of data transformation focused on scaling and formatting data consistently. It involves adjusting values measured on different scales to a common scale, often necessary for comparing and combining datasets.i. Scaling: Techniques such as min-max scaling and z-score normalization adjust data values to a consistent range or distribution, improving the compatibility of data from different sources.
ii. Standardization: Standardization adjusts data to have a mean of zero and a standard deviation of one, which is particularly useful for algorithms that assume data follows a Gaussian distribution.
Conclusion
The integration of Artificial Intelligence (AI) into data analytics offers businesses in different sectors incomparable benefits, thereby presenting a revolutionary concept. The accuracy and efficiency greatly improve decision-making processes AI adds to data analysis, which promotes operational optimization and competitive advantage. Rapid responses to changing market conditions are made possible by real-time data processing, and proactive tactics are made possible by AI's predictive powers. However, the effectiveness of AI-powered analytics depends on high-quality data, which means that careful procedures for data preparation, cleaning, and collection are required. Organizations may seize previously unheard-of opportunities, guaranteeing wise choices and steady growth in a world growing increasingly dependent on data, by adopting AI-powered data analytics and prioritizing managing their data.Engage with expert perspectives, stay informed on advanced developments, and maintain a competitive edge in the rapidly evolving AI landscape with Blue Summit and immerse yourself in the world of AI technology.
REFER TO OTHER RELEVANT CONTENTS
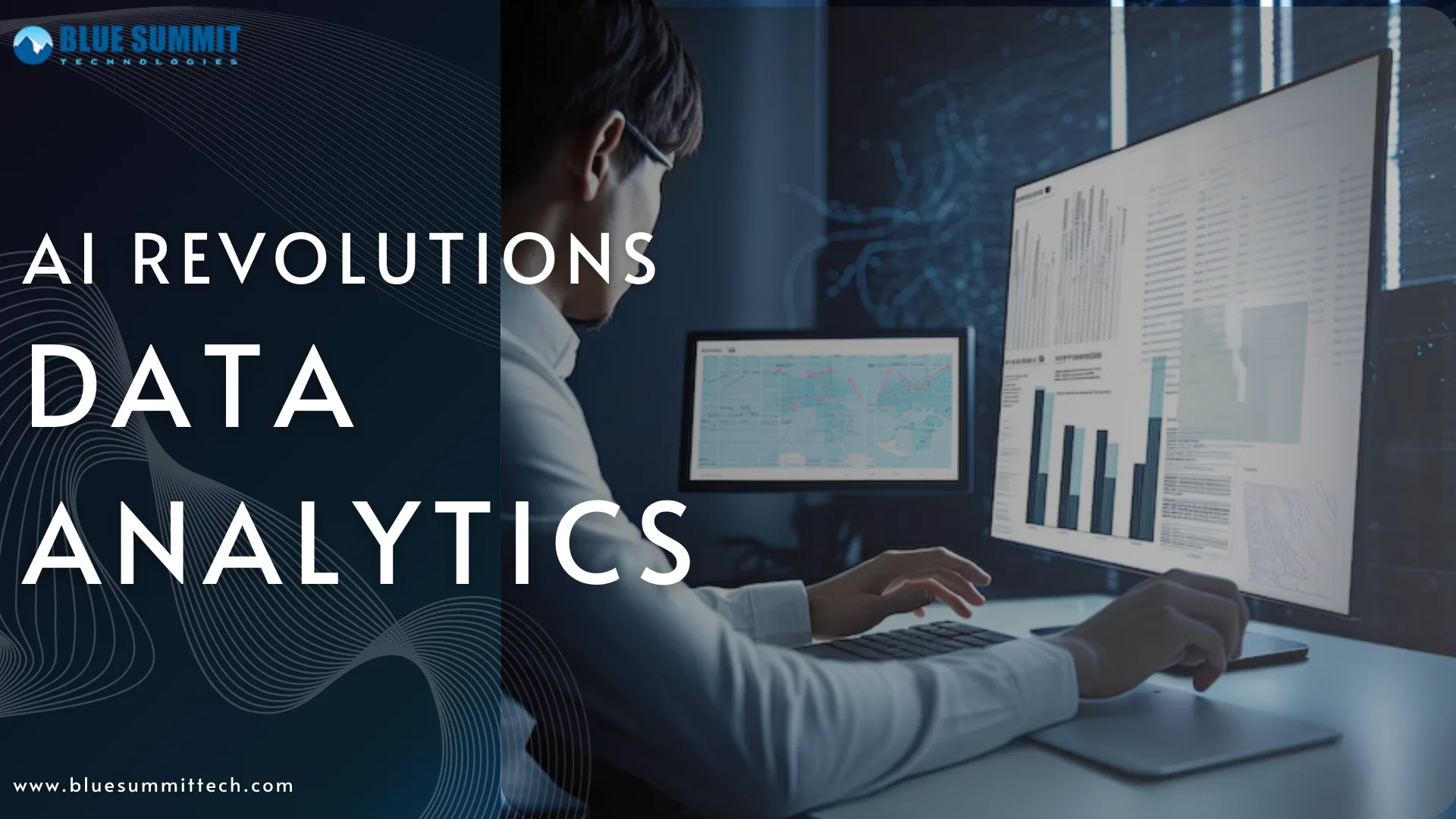
Impact of AI on Data Analytics
Artificial Intelligence (AI) is transforming the field of data analytics, offering significant advancements that improve accuracy, efficiency, and predictive capabilities. Businesses are Using AI for data analysis to automate and optimize data processing tasks, allowing for better decision-making and real-time analysis. Artificial intelligence (AI) is...
read more